How Augmented Analytics Is Redefining Business Data Processing
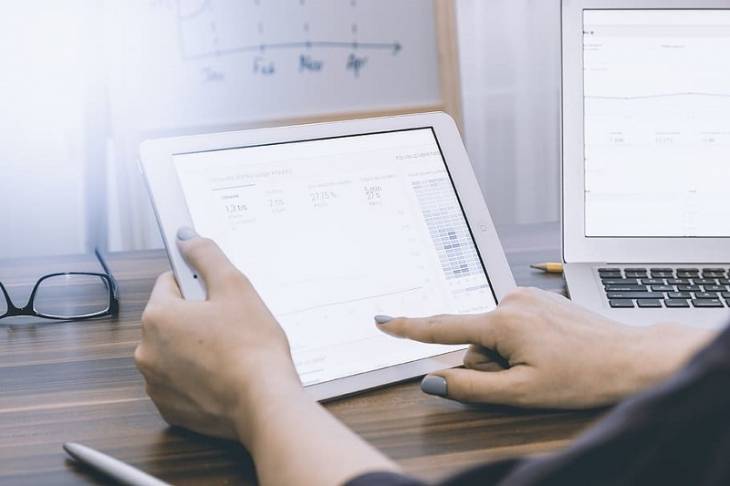
Today’s technology trends are putting more emphasis on data use for business growth and enhanced operations. Each new technology brings with it new opportunities for businesses to improve efficiency, serve customers better, save on expenses among other benefits of technology.
In the quest to apply modern technology effectively in business, however, a lot of data is required, analyzed, and applied for various purposes. The data helps businesses to, among other things, understand their customers better and refine operations.
As such, collecting and analyzing large data sets (Big data) generated in various ways, and applying the insights gained from the data analysis is essential to compete effectively in today’s fiercely competitive business landscape.
Big data, when harnessed and applied correctly using modern tools, can give your business a marked edge for success. The big question, however, is: "How do you harness and draw insights from big data for your business applications' and success?"
It's not so difficult actually.
Harnessing Helpful Business Insights from Data
Many big data analytics tools have emerged and are making it easier than ever before to collect and store business data irrespective of the amount of data in question. Cloud services also meet the requirements for storing data securely, and can also enable easier data processing with multiple features and functionalities that support fast data retrieval.
Moreover, user-generated data also holds a lot of significance today. The data collected from business operations helps in predicting future operations and costs, which give valuable insights. But what matters most is the approach of collecting both those data sets and processing the big data to produce results that can be applied in a business.
An approach for analyzing big data is possible by manually assessing the provided data. Data scientists are required here due to the manual input required for these tasks. However, a newer and simpler method is disrupting this manual approach and redefining business data processing—augmented data analytics and discovery.
According to a 2019 Hype Cycle for Emerging Technologies report by research firm Gartner, new concepts are rising that include advanced augmented data analytics and discovery. Augmented data analytics was previously introduced in Gartner's 2017 report and it entails the addition of machine learning analytics to automate and remove many of the time-consuming manual tasks of data collection and preparation, while ensuring fewer errors.
As augmented analytics and discovery is disrupting and revolutionizing data processing, it is also redefining business success. If you're still wondering how exactly it does that and how you can use augmented analytics to support your own business activities, read on.
Automating Data Processing With Augmented Analytics
Augmented analytics is the use of linguistic and statistical technologies to improve data management, ranging from data analysis to data sharing and business intelligence. It is also entails the ability to transform big data into smaller, more usable, datasets, according to one definition.
Big data analytics is currently more common compared to augmented analytics. In big data analytics, the collected and stored data is analyzed by utilizing machine learning algorithms to produce required outputs and insights from the data. With the help of data scientists, business insights are retrieved from the piles of data collected and put to use.
However, this process is not as easy as it sounds. To connect multiple analytics stages together and choose the best algorithm that can provide the required results, a data scientist has to put in tons of efforts to retrieve results and outcomes that are actually worth using. Hence, without the knowledge of data and its behavior during certain algorithm processing, it is almost impossible to collect the required information and hence, no productive results can be obtained.
It is, therefore, essential to understand the nature of data in your possession and process it accordingly to produce the right results that can actually provide business insights. This is where augmented analytics comes in to improve the analytics process.
Without requiring experts' heavy input and assistance, an augmented analytics system is designed to more easily process and mine data and come up with results that are useful for an enterprise or business, and that provide clear, actionable insights.
Augmented analytics, as defined by Gartner, is basically an approach that aims to automate the processes that provide business insights using machine learning and natural language processing to deliver actionable results. Augmented analytics, thus, disrupts big data analytics by automating tasks such that it is no longer required to invest much human attention.
Leveraging Augmented Analytics Systems
As you may well know, data is everything in today's business and IT world. But processing business data sets and big data to get useful insights is not always easy or a quick process.
Requiring the refinement, filtering, labeling of data, and more means that augmented analytics has to go beyond the standard data processing method to ensure the algorithms process each chunk of data without having to stop in-between.
Therefore, augmented analytics has to follow various steps that are necessary and required to achieve the final desired outcomes in a more effective, efficient, and less labor intensive way.
Key Steps on How Augmented Analytics Works
Here're the key steps on how augmented analytics works you should know if you wish to implement augmented analytics in your business:
Step 1: Preparing the Data for Processing
Before commencing analytics and mining of insights from the collected data, certain refining procedures are needed to convert raw data into the ideal form for processing. This is important even in augmented analytics.
The preparation processes in this step play an important role in acquiring the right outputs as the data is filtered to fit the requirements of further processes to be executed on it. Automation of the data filtering tasks and processes is not yet applied or achieved completely in this stage.
To perform the various operations such as data labeling, data refining, data cleansing, and more, the input of data experts is still required to get the data filtered thoroughly, as even in this inconsistent state the data might have relations that are basically noise only.
Data experts help to clean the data and remove noise that’s present to provide accurate results; that is, useful business insights.
Step 2: Detecting Information from Data
Once the system takes in the input data (refined and cleaned from possible noise), it starts detecting signals that are the product of algorithms implemented on it. The information is detected but the system is yet unable to find the true worth of the detected info. At this stage, experts are required to find useful information and insights that the system is providing.
However, the time taken for experts to analyze and then mine out the information is reduced significantly. The experts look at the results directly instead of laboring on choosing the right algorithm and then executing it. This makes the work of the experts much simpler and easier.
Step 3: Generating Business Insights
The augmented analytics engine is finally ready with outputs; that is, actionable and useful insights. It doesn't require any human intervention to deliver result to the desks of company owners and managers as a direct feed is accessible from the system.
By studying the patterns from past case studies on the business insights, the engine now can suggest appropriate actions based on the generated information and data to provide complete support for business implementation.
For example, in the case of a business that's engagaged in online ride-hailing services and that has recently dropped its market penetration rate, the augmented analytics engine can follow up on the past data and see if there are any actions that caused the change.
If the business is using a taxi app like Uber Clone to support its customers' needs, the engine can check for the features and functionalities that are responsible for bringing more customers. It can then suggest practical, actionable insights that correlate to the penetration ratio decrease. The engine can even track if the actionable insights were put to use or not.
Monitoring the activities and checking for the required results through time is one of the tasks that an augmented analytics system can perform very well and it guarantees excellent results.
Apart from working as an effective substitute or alternative option for a dedicated business analytics team, the augmented analytics powered system can complete many other tasks for a business and provide results that can help the business achieve its goals faster.
Conclusion
Because providing actionable business insights is the primary benefit offered by augmented analytics, businesses can use it to eliminate the possible chances of operational failures and help with understanding market trends better and earlier than the competition.
While not all businesses may think of investing in this technology, there are many benefits offered by the technology that make up for the financial investment it might require.
If you can meet the initial investments in acquiring and implementing augmented analytics system, industry trends have made it easier than ever to use the technology in the day-to-day activities of your business. This also means that implementation of augmented analytics concepts will become increasingly common and affordable for businesses of all types and sizes.
Besides, getting in on new technologies and business approaches early will ultimately lead to more competitiveness in the market and more success for your business.