How Web Scraping Is Helping Businesses Adapt to Downturns
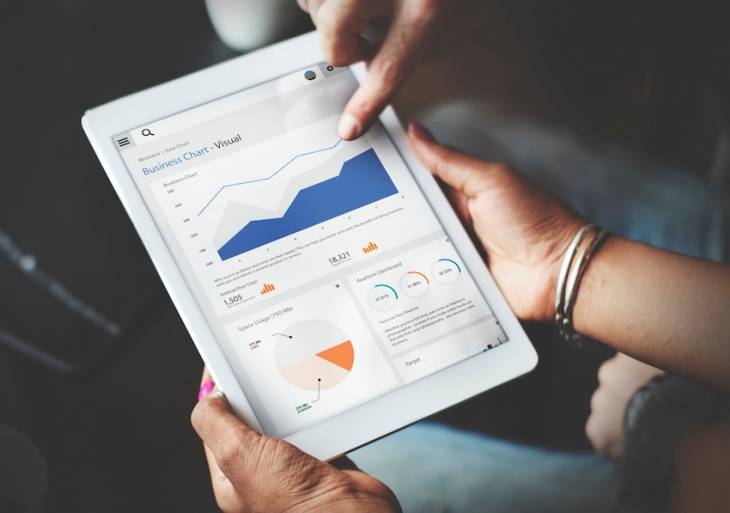
Economic downturns, regardless of the source, affect everyone. Retail and e-commerce businesses are particularly being most sharply affected by current reductions in consumer spending power. Margins in industries are thin, and the value chains across businesses are less flexible regarding pricing.
Businesses are mostly affected by changing consumer behavior. Downturns force consumers to spend less on luxury goods, carefully select essentials, and even reduce spending entirely.
However, ith web scraping, businesses can not only predict changing consumer behavior, but also use the insights drawn from web scraping to strategize better.
But how is this achievable?
A new form of data
Various sources for predicting consumer behavior have been available throughout the years. Many businesses used internal data, such as stock changes, to evaluate how buyers are changing their habits. Unfortunately, internal data is somewhat limited in that it tends to provide a biased view of consumers.
Other sources such as traditional databases or government releases, while providing accurate readings of the consumer, have low publishing rates. As such, they are subject to a form of passthrough effect where once the datasets have been released and analyzed, the insights might already be partly out of date.
Today, however, we have an answer that has none of the issues outlined above – alternative data. Defined as anything that’s not traditional data, it might seem way too broad, but our focus is on the online sphere for now.
Web scraping is the process that uses automated software to collect various publicly available sources of information from nearly any corner of the internet. Be it pricing data from competitors or anonymous internet comments, all of it can be collected in an automated fashion.
Acquiring such data greatly expands our capabilities for building predictive models, especially about consumer behavior. It previously relied, as mentioned, either on internal data or consumer self-reports, both of which have their own set of issues.
Using web scraping to predict changing habits
One aspect of economic downturns and other mishaps we’re all highly familiar with is that everyone becomes significantly more active on various channels. From newly minted experts to regular people, everyone gets involved and shares their thoughts and feelings about the current state of affairs.
These comments pop up everywhere and in large quantities with clear indications of potential changes in behavior. In the current day, rising inflation has been the topic discussed to no end online. Each of these indicates some intention and sentiment towards the changing landscape.
Manually analyzing all of these comments for sentiment and potential indicators for behavioral change would be nearly impossible. Fortunately, natural language processing (NLP) tools have been developed, with giants such as Google offering their solutions for large-scale analysis.
All of these processes, from scraping to sending the data in for NLP analysis, can take only mere minutes, sometimes up to an hour if extremely large volumes are necessary. While collecting a large enough dataset for real world analysis will take slightly more than that, the entire pipeline can be optimized to extremely low lag times.
Alternative data, by itself, might provide useful insights. When combined with internal sources, however, it can show purchasing trends and create predictive power that otherwise would be completely unavailable.
Reorganizing pricing and product selection
Without hard data at hand, it’s hard to predict where consumers will flock to in each downturn. There are some general pointers that I’ve mentioned before as consumers become price-conscious and spend less on luxury goods.
With alternative data and sentiment analysis, however, it becomes much easier to predict how strong these effects will get. Not every economic downturn is equal, so the effects exerted on price-consciousness will differ.
While it may not be possible today, over time a consumer sentiment index can be developed that can be used to understand how powerful the changes in behavior will be. With historical data at hand and post-downturn effects measured, the index could heavily predict areas where and which products and services might be at the greatest risk.
Additionally, even without such an index, ecommerce companies and marketplaces can reorganize stock and warehousing practices to more effectively match changing consumer behavior. Alternative data would allow companies to reduce the costs associated with overstock and revaluation of brands.
Finally, such an approach would benefit consumers in the long run as they would be less affected by supply shocks. During larger disasters, household overstock of non-perishable goods, for example, can lead to a panic-induced spiral as consumers react to, what may seem as, the disappearance of certain goods.
In the end, alternative data enables ecommerce businesses to better prepare for changing conditions globally and, by doing so, they can benefit their buyers as well. With enough effort put into the process, certain aspects of the supply chain, namely the consumer-facing part of it, can become more resilient.