How to Scale Customer Feedback and Learn What Your Customers Really Think
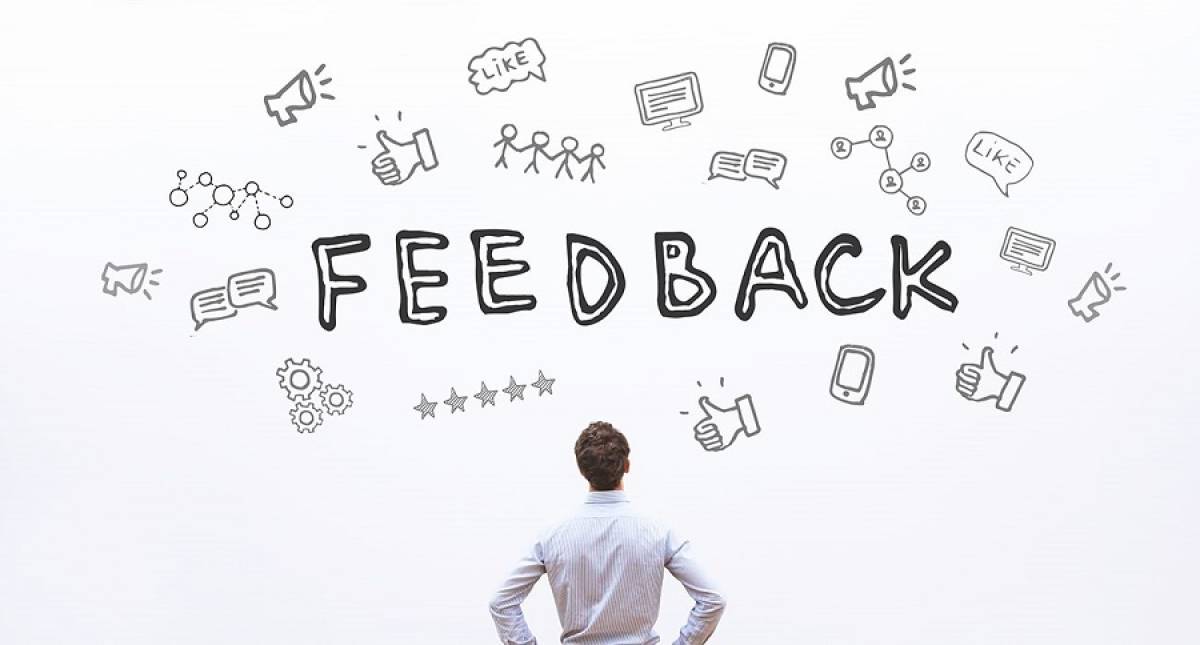
When gathering feedback from your customers, whether it’s through online reviews on platforms like Yelp or Google, social media comments, or survey responses, the most impactful information usually comes in the form of open-ended responses.
It makes sense, a ⅘ rating is important information to have, but it’s even more valuable if the customer explains exactly why they gave you that rating. However, these types of free-form responses are difficult to analyze and compare on a large scale.
If you’re scraping online reviews and gathering survey responses every day, there’s no way your team is going to have time to read every single response. This is where coding qualitative data comes in.
Deductive vs Inductive Coding
To take your qualitative feedback and turn it into easily-measured quantitative metrics, you can follow either deductive or inductive logic. The path you choose usually depends on what stage you’re at in your data collection process.
Deductive coding is best when you already have a scale that you want to use to categorize your data. For example, maybe you’ve already sent out several rounds of customer feedback surveys, and you know you want to measure responses based on a 1-10 scale that rates overall satisfaction with the product. In this case, you would be able to use deductive coding to quickly tag your new data and add it to your existing data set.
Inductive coding is the way to go when you don’t already have a set scale in mind. This is best if you’re analyzing a large amount of qualitative feedback for the first time, so you don’t really know what exactly you’re going to be looking at. Inductive coding is a time-consuming process, but it’s best to spend some extra time getting things right the first time so you can continue to use your measurement scales moving forward.
Once you have established your measurement scale and tagged all of your data, you’ll be able to easily manipulate and analyze your qualitative feedback to identify overarching trends and make changes to your business based on this in-depth information.
Basic Steps for Coding Qualitative Data
Here’s a look at the general process data analysts and customer service teams go through when coding qualitative customer feedback:
Step 1: Assign Categories
The first thing you need to do is decide what category or facet of your business the review is concerned with. For example, this could be about the price of the product, the quality of the product, the level of service, or any other number of factors.
Depending on your industry, you can break these up further into satisfaction levels for a number of different products or business locations.
Step 2: Assign Sentiments
Now, take that same review and decide what the overall sentiment is. Is it very satisfied? Very angry? Pretty neutral? Once you’ve categorized a number of pieces of feedback, you’ll likely start to see trends and patterns that will help you come up with your measuring scale.
Step 3: Combine Categories and Sentiments
This seems pretty straightforward, right? The next step is to combine the categories and sentiments of each piece of feedback so that you’re able to get an overall satisfaction view of that particular service.
For example, you may notice that 70% of reviews concerning your product price fall into the “very negative” sentiment. That’s a pretty clear indication that something needs to change with your pricing structure.
How AI Can Help Speed Up Customer Feedback Analysis - Infographic
These days, businesses are lucky that there are so many AI-powered analysis tools available. These types of programs can actually sort through the data on their own, either abiding by a predetermined set of criteria or even identifying new patterns.
It costs a lot of employee bandwidth to manually comb through all pieces of customer feedback, so many business are opting to have bots lend a helping hand and take up most of the grunt work.
It can be hard to visualize these practices just by reading about them, so the infographic below by Chattermill should shed more light on this topic by providing specific examples: