Ethics of Machine Learning: Balancing Innovation with Responsibility
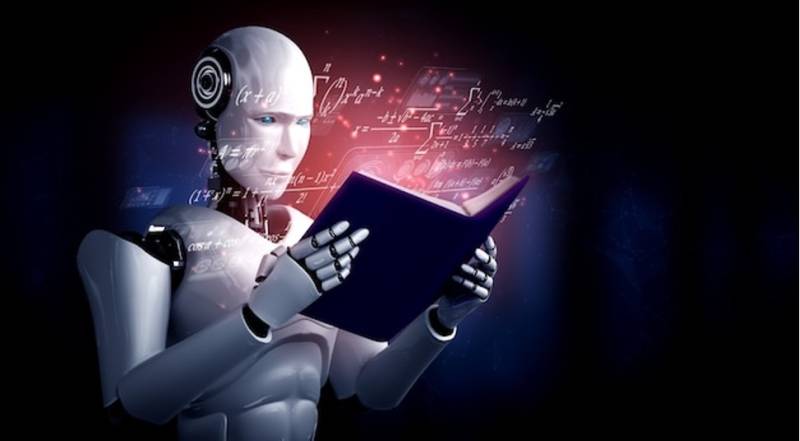
As we move deeper into a more technology-driven world, the applications of machine learning have become increasingly common and useful in various industries. From healthcare to finance, transportation and retail, machine learning algorithms are transforming the way we live, work and interact with the world around us.
However, with great innovation comes great responsibility; and the ethics of machine learning (ML) have become a topic of concern for many individuals and organizations alike. In this article, we will explore the ethics of machine learning and importance of balancing innovation with responsibility.
We will discuss the potential benefits and risks associated with ML, the current state of ethical considerations in the field, and what we can do to ensure machine learning remains a force for good.
But, before diving into the ethics of machine learning, let's first define what we mean by the term.
What Is Machine Learning?
Machine learning is a subfield of artificial intelligence that uses algorithms and statistical models to enable computers to learn from data without being explicitly programmed. In other words, machine learning algorithms are designed to learn from patterns in data and make predictions or decisions based on that learning.
One of the most significant advantages of machine learning is its ability to analyze vast amounts of data quickly and accurately. This has made it an essential tool in industries where data analysis is critical, such as healthcare and finance.
Machine learning algorithms can help identify trends and patterns that humans may not be able to detect, leading to improved diagnosis, treatment, and financial decision-making.
Benefits of Machine Learning
The potential benefits of machine learning are numerous and significant. A few examples include:
- Improved efficiency: Machine learning algorithms can automate tasks and processes that would otherwise require human labor, resulting in increased efficiency and cost savings.
- Better decision-making: Machine learning algorithms can analyze data quickly and accurately, providing insights that can lead to better decision-making in fields such as finance and healthcare.
- Improved customer experience: Machine learning algorithms can help businesses personalize their services to individual customers, resulting in a better customer experience and increased loyalty.
- Improved safety: Machine learning algorithms can be used to detect potential safety issues in industries such as transportation and healthcare, leading to improved safety and reduced risk.
Risks of Machine Learning
While the potential benefits of machine learning are significant, there are also risks associated with the technology. Some examples of ML risks are:
- Bias: Machine learning algorithms can be biased based on the data they are trained on. If the data contains biases, the algorithm will learn and replicate those biases, leading to unfair or discriminatory outcomes.
- Privacy: Machine learning algorithms often require access to large amounts of data, which can raise privacy concerns. If the data is not properly protected, it could be accessed by unauthorized individuals or used for unintended purposes.
- Security: Machine learning algorithms can be vulnerable to cyberattacks, leading to potential breaches of sensitive data or system failures.
- Accountability: Machine learning algorithms can make decisions that have significant consequences, but it can be challenging to determine who is responsible for those decisions.
Ethical Considerations in Machine Learning
Given the potential benefits and risks associated with machine learning, it is essential to consider the ethical implications of the technology. Some key ethical considerations include:
- Fairness and Bias: It is essential to ensure that machine learning algorithms are fair and unbiased. This requires ensuring that the data used to train the algorithm is representative and does not contain biases. Additionally, algorithms should be monitored for bias regularly.
- Transparency: Machine learning algorithms can be opaque, making it challenging to understand how decisions are being made. Ensuring that algorithms are transparent and explainable can help build trust in the technology.
- Privacy: Protecting the privacy of individuals whose data is being used by machine learning algorithms is critical. This requires implementing appropriate data protection measures and ensuring that individuals are informed about how their data is being used.
- Accountability: Ensuring accountability for the decisions made by machine learning algorithms is critical. This may involve identifying who is responsible for decisions made by the algorithm and ensuring that they can be held accountable for any negative consequences.
- Social Impact: Machine learning algorithms can have significant social impacts, and it is essential to consider these impacts when designing and implementing the technology. This requires considering how the technology will affect different groups of people and ensuring that it is used in a way that benefits society as a whole.
- Human Oversight: While machine learning algorithms can automate many tasks, it is still important to have human oversight to ensure that decisions made by the algorithm align with ethical and moral standards.
- Continuous Improvement: The development of machine learning algorithms should be an ongoing process that includes regular reviews and updates to ensure that the technology is working as intended and continues to align with ethical considerations.
Balancing Innovation with Responsibility
As we have seen, the ethics of machine learning are complex and multifaceted. While there is significant potential for machine learning to transform industries and improve our lives, it is essential to balance innovation with responsibility to ensure that the technology is used ethically. This requires a collaborative effort between individuals, organizations, and policymakers.
Individuals should take the time to educate themselves about the ethical implications of machine learning and advocate for responsible development and use of the technology.
Organizations should prioritize ethical considerations when designing and implementing machine learning algorithms and ensure that they are transparent about how the technology is being used.
Policymakers should work to establish regulatory frameworks that ensure the responsible development and use of machine learning.
Conclusion
The ethics of machine learning are critical to ensuring that the technology is used in a way that benefits society as a whole.
While the potential benefits of machine learning are significant, there are also risks associated with the technology that must be considered.
By prioritizing ethical considerations and working collaboratively to ensure responsible development and use of machine learning, we can ensure that the technology is a force for good.